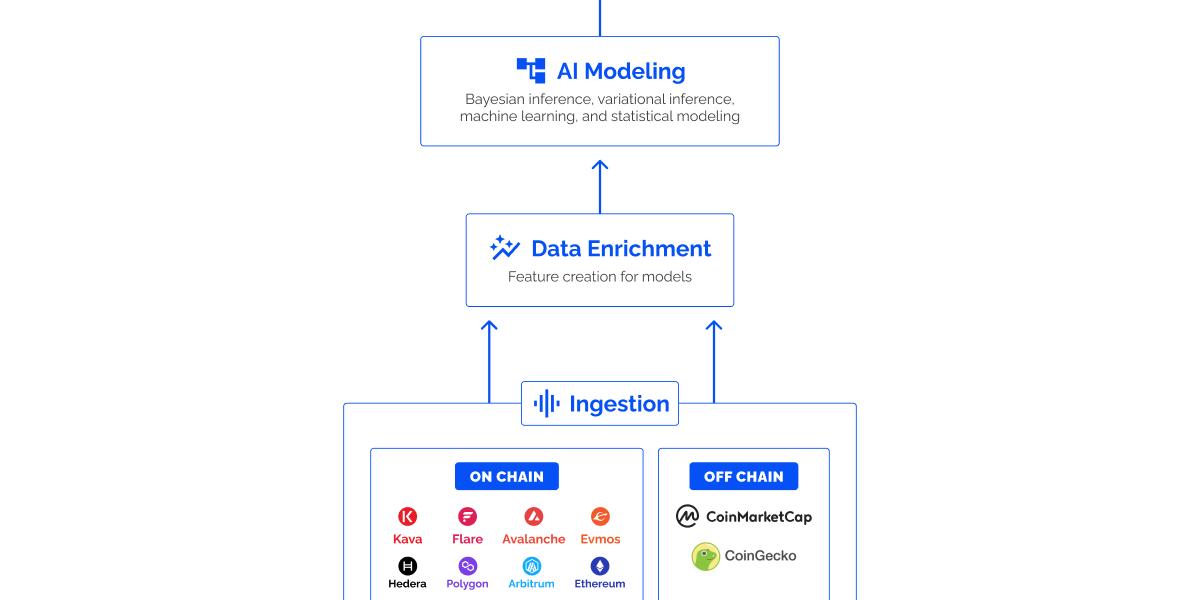
White Paper
AI Risk Modeling: The DEX Use Case
July 11, 2024
Background
The advent of blockchain technology and the emergence of decentralized finance (DeFi) has revolutionized the traditional financial landscape by introducing a more open, transparent, and accessible financial system. This transformation has enabled individuals worldwide to engage in financial activities without relying on traditional banks or intermediaries, thereby reducing costs and increasing efficiency. As a result, DeFi has the potential to foster financial inclusion, innovation, and economic growth, challenging the established norms of the global financial system.
At the same time, each innovative DeFi application introduces new financial risk management challenges. In traditional finance (TradFi), such products have significant operational history, are highly regulated, and are supported by teams of financial professionals dedicated to managing financial risk. In contrast, the emerging nature of DeFi results in a wide range of vulnerabilities due to its relatively more limited operational track record, new technology tools and techniques and in many cases fundamentally new and unregulated business models..
At Ledger Works, we bridge the gap between DeFi innovation and effective risk management by leveraging AI, machine learning, and statistical modeling to develop DeFi specific risk management solutions.
Inference Lifecycle
In the evolving landscape of DeFi, understanding and managing risk is crucial. The graphic in Appendix I illustrates the lifecycle of the Ledger Works inference platform, designed to comprehensively address this need by implementing fully integrated on-chain and off-chain processing. Data is ingested in near real-time directly from on-chain nodes and off-chain providers, ensuring a robust foundation of information-rich features for analytics and model development. This comprehensive data collection supports the training of production-ready models, which are subsequently used to continually run inferences leveraging the lower cost and higher efficiency of data and compute off-chain. The outputs of these models are persisted and seamlessly integrated back on-chain into smart contracts and bots, enabling them to adapt their behavior in real time.
Use Case: Liquidity Provider Fee Optimization
As decentralized exchanges (DEXs) and their underlying automated market-making (AMM) algorithms evolve, so too must the sophistication of the tools necessary to manage their transaction fees. From static and relatively simple fee models, DEXs are increasingly looking to leverage more dynamic fee maximizing approaches.
To design models to effectively maximize fees, DEXs require a hybrid that leverages real-time on-chain data, combined with the computational power of off-chain ML based models to support the implementation of a dynamic fee maximizing system. These dynamic fees allow protocols to adjust charges on individual trades to better align with their objectives. To address the issue of “when” and “by how much”, Ledger Works has developed a Bayesian inference model that dynamically adjusts fees based on statistics derived from the posterior distribution of the AMMs underlying asset returns. These dynamic fee recommendations are then used to update on-chain protocol fee amounts.
This sophisticated model is further extended to include a Monte Carlo simulation within the same Bayesian framework. The simulation provides a detailed analysis of the posterior distributions of off-chain asset price returns, on-chain Total Value Locked (TVL), liquidity, and volume for individual AMMs, offering a comprehensive forecast of protocol revenue and the expected return for the liquidity provider.
By integrating these advanced models, DeFi protocols can effectively optimize revenue and enhance liquidity provider fees, all while managing the inherent risks of the decentralized financial landscape. With these capabilities, Ledger Works empowers clients to leverage off-chain compute and data driven solutions, to make on-chain decisions that enhance operational efficiency and drive sustainable growth.
Appendix I: Inference Platform Design
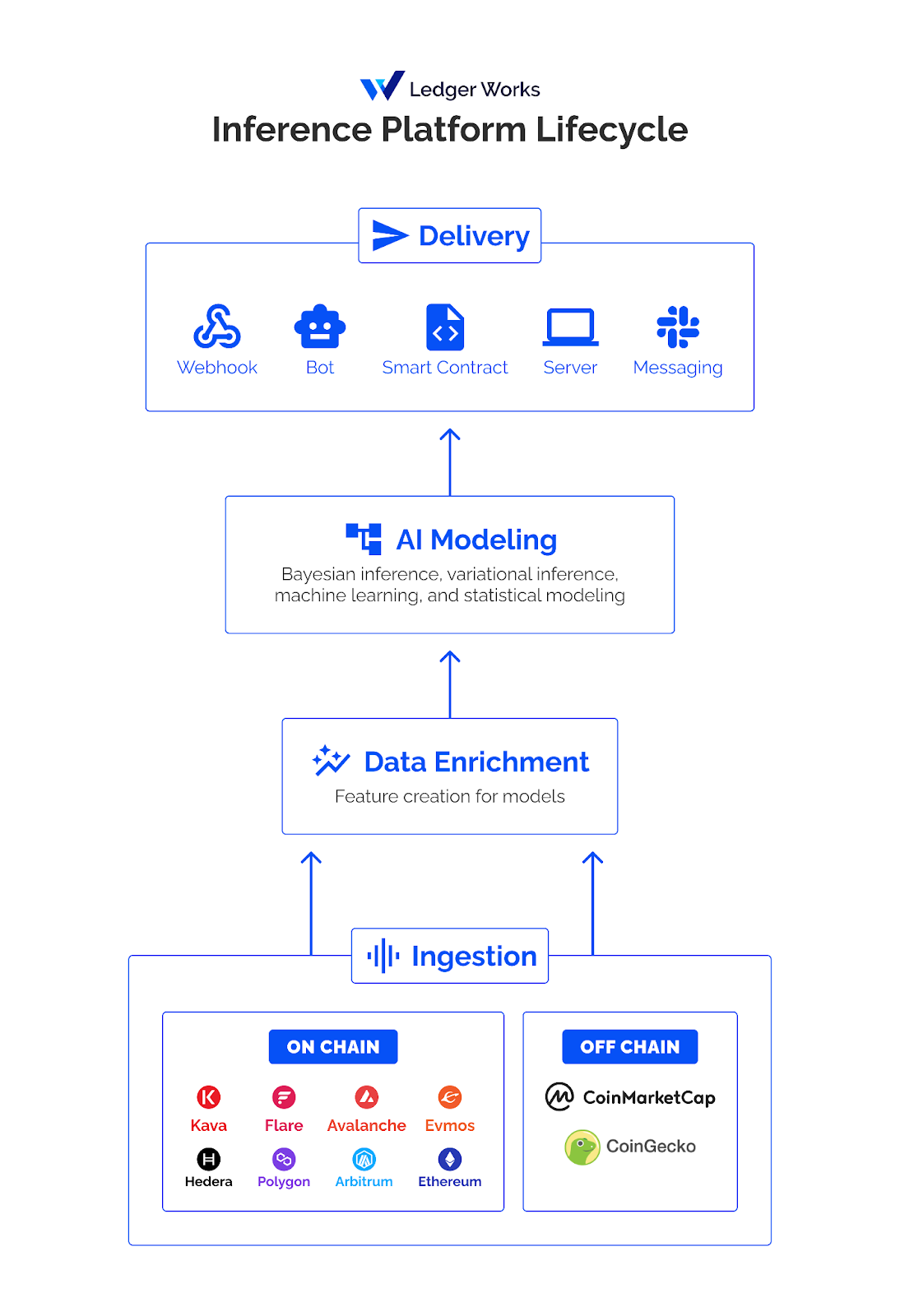